Global supply chains have been disrupted in recent years due to geopolitical changes, pandemic response, energy crises and other factors. Manufacturers need to redevelop their production and supply chains, leveraging CEI, automation and related technologies to increase productivity, flexibility and resilience.
The following “Industry Spotlight Use Cases” sections are curated based on comprehensive surveys conducted by IDC in 2023, targeting market and industry professionals across Europe. The examples accompanying each spotlight use case category are derived from use cases executed by the Research and Innovation Action teams within the EUCloudEdgeIoT community. As research progresses, we will continue to update this information to reflect the latest findings and developments.
Spotlight use case categories
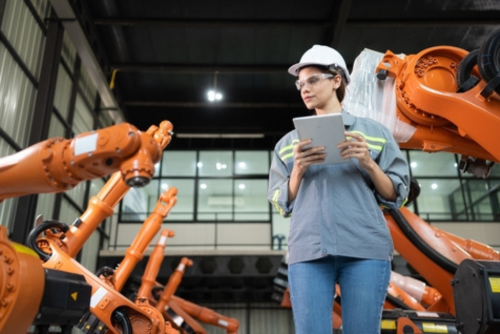
Asset monitoring and maintenance
Manufacturers' equipment requires regular monitoring and maintenance, which often involves significant labour. Many operations systems have long lifespans and do not yet employ the latest IoT systems. Many manufacturers still require employees to visually inspect gauges at various machines and log the data to detect anomalies. To modernize these systems, manufacturers can install asset monitoring systems to detect such conditions as temperature, vibration, moisture, chemical exposure, pressure, and others, to provide real-time visibility into their asset conditions. These systems can then identify changes or problems and initiate maintenance. By doing so, this solution reduces costs, extends the life of equipment, reduces errors, increases productivity and reduces downtime. Such systems can also be integrated into digital twins and integrated with other operations and IT systems for easier management, increased efficiency and moves toward automation. It is the most widely used use case in manufacturing, used by 64% of respondents in the industry. it is highly accessible and often one of the first use cases to be adopted. It can also be a foundation for larger CEI/digitalization initiatives that bring large gains to the industry. Because manufacturers typically have substantial operations and IT assets concentrated on-premises, they also tend to have local staff qualified to deploy and maintain these systems. As a result, they are good candidates to use significant edge computing. Asset monitoring can include functions for emergency response and shutdowns that require real-time response, the highest reliability and the lowest latency, which points to using some form of edge computing. However, much of the asset monitoring can also be less critical and quite comfortably utilize cloud computing. As such, this use case will likely use a mix of edge and cloud infrastructure.
Sub-category use-cases
- Asset tracking
- Condition monitoring for temperature, vibration, pressure and other factors
- Predictive maintenance
- Chemical sensors for employee safety
- Leak detection systems
- Emergency response
- Operations system automation
Projects use-cases
Data-driven Cognitive Production Lines
The evolution of existing modular manufacturing systems needs strategies towards mass customisation for processing a wide range of products.
The fast use and processing of data is of paramount importance to make intelligent, automated, human-centred augmented and assisted decisions.
aerOS will introduce the enablers to raise production autonomy as its distributed edge-powered modular approach will facilitate IoT edge-cloud continuity.
Objectives and expected benefits:
- First worldwide autonomous production line (Level 4) introducing an open modular edge orchestration approach/OS building on IoT, Big
- Data & ROS2 communities.
- Quality, circularity, zero footprint and AI testing and validation.
- Effective data distribution and sharing closer to the source, ensuring data integrity and security.
Safe human/machine collaboration. - Dynamic intralogistics adaptation and autonomous production scheduling

Automated Guided Vehicles (AGVs) and Robots
Factories, warehouses and logistics centres feature many moving parts and inventory. Forklifts and order fulfilment tend to require significant labour, with employees navigating facilities amidst large equipment, creating safety risks. AGVs and robots enable the automation of many of these processes. Items can be transported throughout the facilities without requiring a worker’s attention and without putting workers at risk. As such, these solutions can increase efficiency, reduce accidents and increase safety. It is still nascent and requires extensive investment and innovation over the next decade to reach its potential. AGVs and robots require extensive computing resources in close proximity to ensure reliability and very low latency. As are likely to rely heavily on edge computing. Many companies expect 5G networks to play a critical role in enabling this use case, as 5G networks will gradually enable extreme reliability and low latency. In many cases, companies are working with private 5G networks to provide that connectivity within their facilities. Additionally, these solutions are still likely to integrate some cloud computing for broader management and analytics.
Sub-category use-cases
- Automated guided vehicles
- Automated forklifts
- Vehicle routing
- Autonomous mobile robots
- Item pickers
- Automated freight loading/unloading
- Delivering supplies to staging areas
- Assembly line robots
- Cobots
- Warehouse automation
- Worker support systems
- Inventory management systems
Projects use-cases
Robotics, particularly Cobots, have a strong impact within highly automated production facilities. As market demands transformation and demographic change induce new challenges on mass production, Continental investigates Cobots and AGVs with respect to the strict requirements of electronic production.
This pilot takes place in one of the Continental plants, which will test some of NEMO’s capabilities in Innovative manufacturing processes.
Objectives and expected benefits
Combine 3D Vision, heterogeneous networks, edge (remote) and semi-autonomous (on-device) CF-DRL/ Transfer Learning, enabling fully automated SMD-Components recognition and transfer to the production site
AGVs-AGVs and AGVs-humans collision prediction, detection and avoidance through real-time cm-level High Accuracy Indoor Positioning (HAIP) and CF-DRL functions hosted locally on the IoT nodes and in 5G edge.
Digital powertrain optimisation (production, process, asset and energy) in brownfield factories
Validate NEMO user acceptance from an industry employee viewpoint by validating efficiency and productivity

Visual Inspection and Quality Control
Quality control is a crucial step in production, but it poses challenges. It requires human attention, possibly in hard-to-reach locations, and errors may be missed. Using cameras and AI-based analytics, many quality control functions may be automated, such as identifying problems in welds or ensuring that the right components are installed to meet specifications. As such, this use case can increase efficiency, reduce waste, and improve customer satisfaction. It also uses AI-based imagery analysis, which is a core component of many other use cases. it is one of the most commonly used use cases in manufacturing. It was the third-most common use case listed in the survey, used by 59% of respondents. Automobile manufacturers also mention it as a key IoT use case they are using to achieve significant gains at a modest cost. This use case is also of interest because it utilises AI-based imagery analysis, which is becoming a core component of many different use cases. Therefore, it is useful to examine this use case in detail to see what lessons it might hold for similar use cases leveraging AI-based imagery analysis in other areas. To manage this solution, cameras collect images of various components and features, and AI-based analytics models analyse the data to detect whether it complies with requirements. If not, it can alert that changes are needed. The images may be analysed on local edge computing to reduce the amount of data traversing the network. However, this use case may not be critically dependent on real-time analytics, and some solutions send the data to the cloud for analysis. Cloud resources are also helpful in coordinating across locations and for training and managing the analytics models.
Sub-category use-cases
- AI analysis of medical images and scans
- AI recommendation engine for treatments
- Data/imagery management systems for training AI models, deploying them to the edge and updating them as AI models improve